Antonio Sclocchi
Research Fellow, Gatsby Unit, UCL Theory of Learning Lab
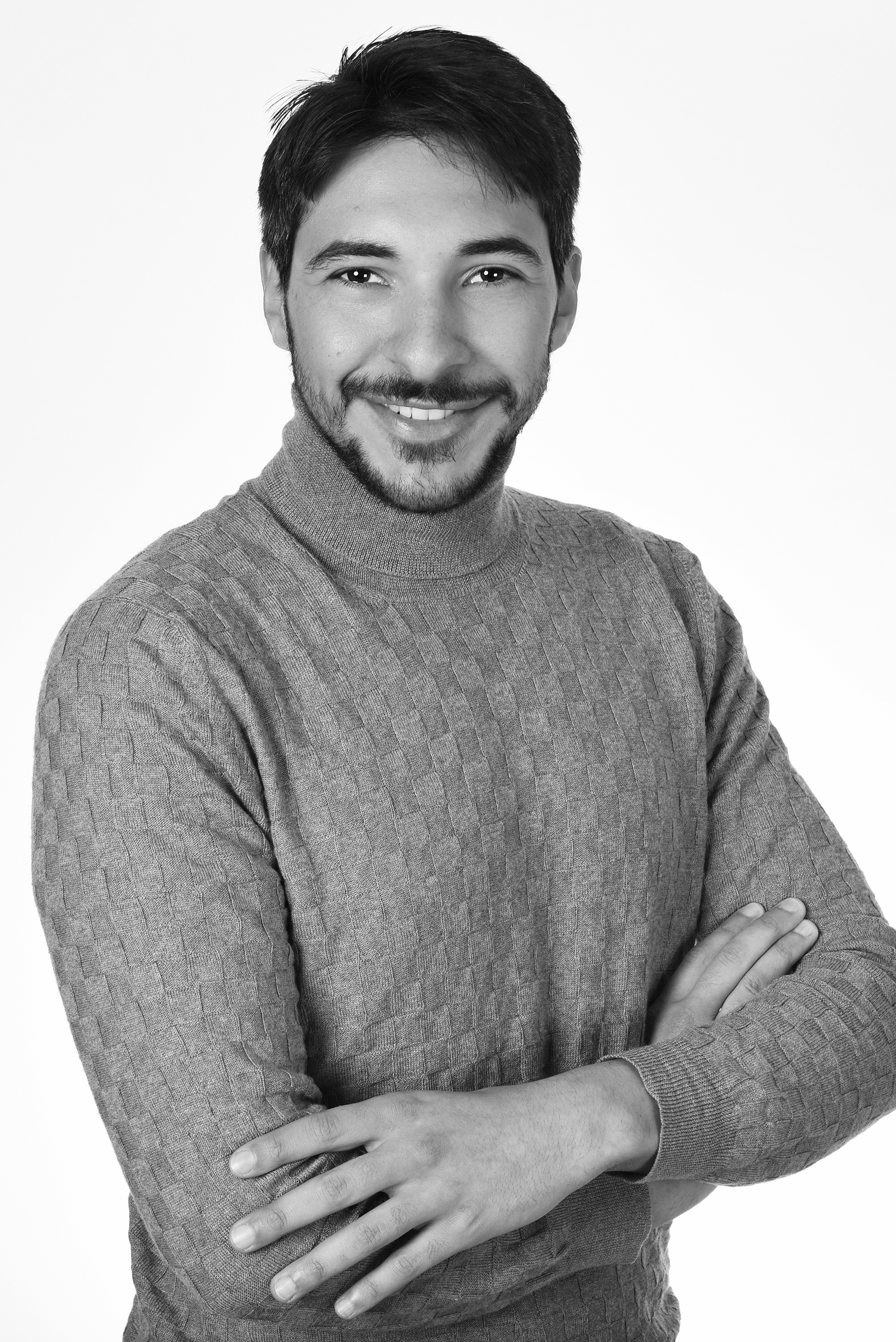
Sainsbury Wellcome Centre, UCL
25 Howland St
London, UK
I am a theoretical physicist working at the intersection between statistical physics and deep learning. My current research interest revolves around neural network optimization, diffusion models, and data structure.
Currently, I work in the group of Andrew Saxe at UCL. Previously, I worked in the group of Matthieu Wyart at EPFL, building models of data structure and learning dynamics inspired by statistical physics. I earned a PhD in theoretical physics at LPTMS, Université Paris-Saclay under the supervision of Silvio Franz and Pierfrancesco Urbani, working on disordered and glassy systems.
recent publications
- How compositional generalization and creativity improve as diffusion models are trained2025
- Probing the Latent Hierarchical Structure of Data via Diffusion ModelsIn The Thirteenth International Conference on Learning Representations , 2025
recent news
Feb 17, 2025 | Our work on compositional generalization and creativity just appeared on the arXiv |
---|---|
Jan 22, 2025 | Our work on probing the data structure with diffusion models has been accepted at ICLR 2025 ![]() |
Jan 02, 2025 | Our work on diffusion models on hierarchical data has been finally published on PNAS ![]() |
Feb 20, 2024 | Our work on Stochastic Gradient Descent has been finally published on PNAS. |